The Ultimate Guide to AI and Machine Learning: Differences, Benefits, and Implementation Strategies
As the world moves towards digital transformation, artificial intelligence (AI) and machine learning (ML) have emerged as transcendent solutions, propelling businesses into the future. The capabilities of AI can quickly process large volumes of data, and ML interacts with those data to extract patterns and make predictions.
These technologies change how businesses operate and drive efficiency and innovation across industries.
This comprehensive guide equips you with practical knowledge of AI and machine learning solutions, encompassing their differences, benefits, and strategies for implementing these technologies. By the end, you’ll be ready to leverage the power of AI and ML in your business.
Artificial Intelligence
1. What is Artificial Intelligence?
Artificial intelligence, or AI, is an emerging technology that simulates human intelligence, performs problem-solving and reasoning, and automates routine tasks. It is achieved through analysing data and making decisions based on patterns and rules, enhancing efficiency and accuracy in various applications.
AI is used in everyday life; you might not notice that Siri, the AI virtual assistant, is the best example. It helps set reminders, answer questions, schedule appointments, and provide weather updates.
AI-powered solutions enhance convenience through voice commands, making information more accessible and simplifying everyday interactions.
“Simply put, artificial intelligence (AI) is a branch of computer science that creates systems that think like humans.
AI is applied in every industry and business function, like accounting, customer service, cybersecurity, IT operations, financial analysis, HR, legal, sales, marketing, and supply chain management.
2. How will AI help businesses?
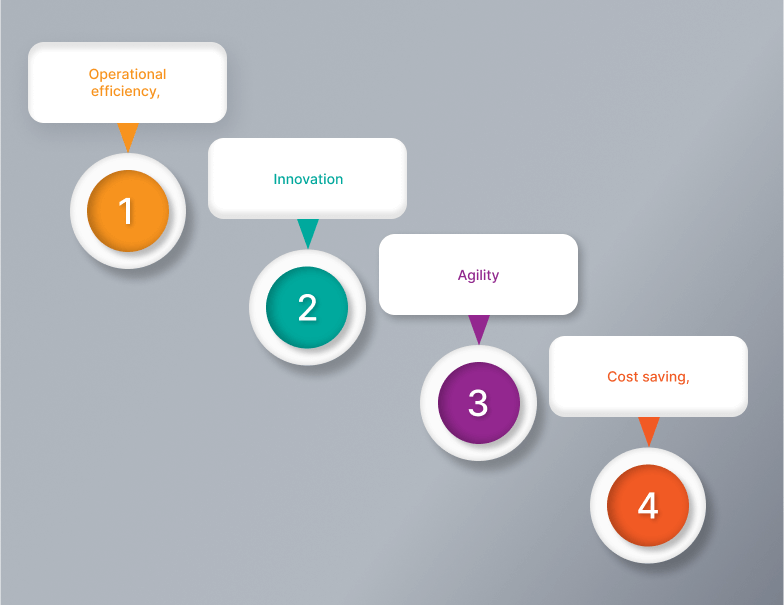
Businesses greatly benefit from AI through
- Operational efficiency
- Innovation
- Agility
- Cost saving
Besides, artificial intelligence solutions foster business operations by enabling informed decisions, improving accuracy, and driving personalised campaigns, which can lead to higher conversion rates.
For example, conversational bots are an ongoing innovation and the best example of AI. Organisations leverage this technology in their customer support systems to answer customers’ questions about products or services and provide instant, accurate responses to common queries.
This approach frees human intervention from complex issues and analyses feedback to improve products and customer satisfaction.
3. Types of AI
Distinctive types of AI serve a unique approach based on their capabilities and functions in technology and industry.
The three types of AI include:
- Narrow AI: Narrow AI, also known as simple AI or specific AI, focuses on performing specific or limited tasks more efficiently. Its knowledge is not transferable to other domains due to its restricted scope of programmed functionalities and limited comprehension of knowledge.
Examples: Image and speech recognition, Search Engines. - General AI: General intelligence has a broad spectrum of understanding and capabilities similar to humans. It performs intellectual tasks, self-teaches, and solves complex problems.
Examples: Self-driving cars - Super Intelligence AI: Superintelligence, often referred to as the future of AI, has the potential to surpass human cognitive abilities, which not only perform human tasks but also integrate with human emotions. However, it also poses a significant threat that requires extensive training.
Examples: Humanoid robots
4. Benefits of AI
- Intelligent Decision Making : AI analyses vast amounts of data quickly, providing actionable insights for better decision-making. This results in more strategic planning and problem-solving capabilities. Its ability to simulate scenarios ensures optimised resource allocation, reduces human error and stimulates proactive decisions
- Improved Accuracy : AI technology guarantees greater data integrity and reliability by significantly decreasing human error, especially in data entry and analysis jobs. This provides enterprises with more precise financial reporting, enhanced regulatory compliance, and better decision-making procedures.
- Personalisation : AI enables businesses to create tailored customer experiences, adapting communications based on individual preferences. By analysing user data, AI tailors customer interaction and fosters deeper customer engagement.
- Improved Safety : AI algorithms detect and mitigate potential risks and challenges, including security breaches and cybersecurity hazards, to foster safe work environments.
- 24/7 Availability : With the help of AI chatbots and virtual assistants, businesses can offer streamlined customer support throughout the day and immediate assistance to boost customer satisfaction.
- Scalability : Businesses leverage AI to scale operations to meet growing business demands and perform day-to-day activities. AI solutions can handle increased data volumes and complexity without compromising performance, allowing companies to expand while maintaining efficiency and cost-effectiveness.
Machine Learning (ML)
1. What is Machine Learning?
Machine learning (ML) is a branch of AI focused on building algorithms that allow systems to learn from big data and analyse it independently. It leverages algorithms and statistical models to interpret complex data patterns, enabling intelligent automation and decision-making.
Over time, ML algorithms independently make decisions based on training data, which is gathered, prepared, and used to train the model, gradually yielding business results.
2. How is Machine Learning Helpful for Business?
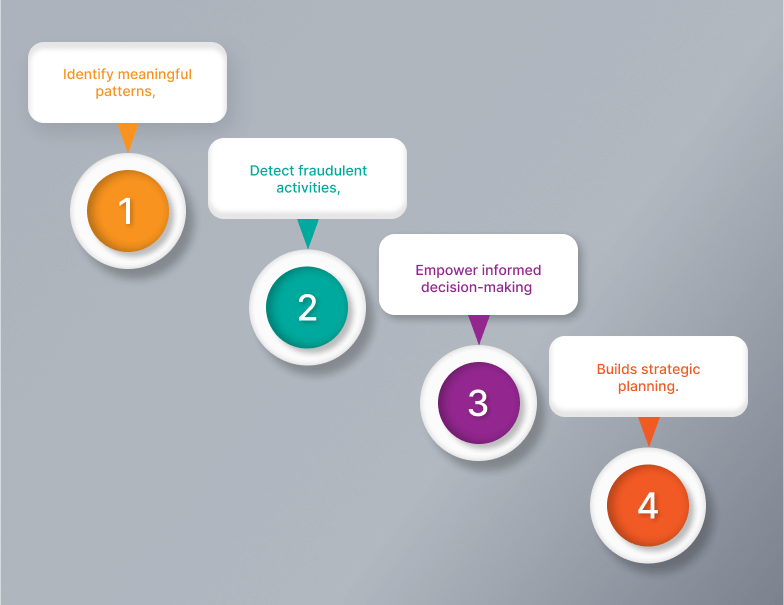
Employing machine learning in businesses enhances efficiency and fosters innovation. By analysing raw data from various sources, ML algorithms transform data into actionable intelligence.
They provide significant insights that help businesses
- Identify meaningful patterns
- Detect fraudulent activities
- Empower informed decision-making
- Builds strategic planning.
Machine learning predicts future trends and customer behaviours, allowing businesses to tailor their offerings effectively and mitigate risks and fraud.
For example, ML is a critical asset for healthcare. It is integrated with X-rays and magnetic resonance imaging (MRIs) to diagnose health issues accurately. The ML algorithm analyses digital medical records to identify risks for specific conditions, enabling timely and personalised treatments that improve patient outcomes.
3. Types of Machine Learning
Machine learning algorithms leverage and analyse data involving historical and past events to predict the future. They help businesses solve complex problems involving regression, classification, forecasting, clustering, and associations.
It is clear that ML is a training engine that builds algorithms based on past experience, which is not applicable to every task.
Machine learning types are:
- Supervised Machine Learning : This type of machine learning is based only on supervision, and the ML model is trained only on labelled datasets. Labelled datasets have input with known outputs, which are used to train a machine to predict outputs for new data.
Examples: In the financial industry, a supervised ML model spots credit card fraud by studying labelled transaction data. It learns to detect unusual patterns that signal fraud, improves with new data, and adjusts to new fraud strategies. - Unsupervised Machine Learning: This ML model is trained on unlabeled datasets that avoid supervision. Unlabeled datasets contain data lacking predefined categories or indicating specific outcomes. The algorithm’s primary role is to identify patterns and relationships within the data and group unsorted datasets.
Examples: An unsupervised ML algorithm segments customers by analysing their purchasing patterns without predefined categories in the retail industry. It helps businesses understand distinct customer groups and tailor marketing strategies accordingly.
- Semi-Supervised Learning: Semi-supervised emphasises clearly that this learning model combines supervised and unsupervised machine learning. This ML algorithm is trained with both labelled and unlabeled datasets. It is also highly efficient and solves the drawbacks and challenges of those learning models.
Examples: A semi-supervised model in image recognition involves using limited labelled datasets to predict objects in large datasets of unlabeled images. This approach helps the model understand the similarities among objects in labelled images throughout the dataset, making its predictions more accurate while handling many photos. - Reinforcement Learning: This machine-learning model works based on feedback initiatives, where it interacts with its surroundings to learn patterns and behaviours. With the trial-and-error method, actions are produced and learned from events, and performance is improved for better outcomes.
Examples: Cobot arms in manufacturing perform production activities based on programming and predefined algorithms. It is also called robotic arms that learn to perform tasks more efficiently, adjusting their actions based on feedback from sensors, improving production speed, and reducing errors.
4. Benefits of Machine Learning
- Predictive Analytics: By leveraging machine learning solutions, businesses can predict future events, customer behaviours, and market shifts. This enables businesses to make better decisions, allocate resources, and target marketing campaigns that drive higher profitability and customer satisfaction.
- Enhanced Cybersecurity: ML algorithms can process large amounts of data and predict critical events in real-time. With previous datasets, ML models can detect threats in the early phases and uncover network vulnerabilities.
Also, ML automates tasks like updating and monitoring activities and reduces IT workload and costs, which is crucial for businesses managing cyber risks effectively.</span< - Fraud Detection: Machine learning (ML) identifies unusual patterns in customer transactions, significantly enhancing the accuracy and efficiency of fraud detection systems. With continuous learning and adaptation, ML algorithms train and improve their ability to detect complicated fraud activities. This proactive approach helps businesses minimise financial losses and protect customer trust, ensuring robust security.
- Data Mining: Machine learning algorithms extract intricate patterns and correlations from extensive datasets to streamline data mining. This capability enhances operational efficiency through valuable insights from large datasets to identify trends, enhance operations, and predict customer behaviours.
This approach results in cost savings and improved service quality. - Improved User Experience: ML enhances user experiences by tailoring product recommendations and content based on previous interactions. This effectively boosts user engagement and fosters long-term retention. It also ensures that users receive relevant sources, creating a more satisfying and intuitive user journey.
- Competitive Edge: Integrating ML into business strategies provides a significant competitive advantage by improving decision-making and operational efficiency. ML algorithms adapt to new data, allowing businesses to respond quickly to market changes and stay ahead of competitors.
Through its benefits, machine learning helps fuel business innovation and empowers the creation of new products, services, and customer experiences.
Business Consequences of Avoiding AI and ML Solutions
Leveraging AI and ML solutions offers enterprises a range of benefits, but it is necessary to understand the drawbacks of not using these technologies.
1. Difficulty in Forecasting Business Opportunities
Traditional forecasting methods fail to handle complex datasets, resulting in unreliable business predictions and an increased risk of making uninformed decisions.
Solution: Deploy AI and ML-driven models to continuously learn and adapt, offering real-time insights and future scenario simulations to guide business strategies effectively.
2. Poor Data Quality
Without AI and ML, data management becomes more challenging, leading to errors and inconsistencies that compromise the integrity of business insights.
Solution: Implementing machine learning techniques can minimise inconsistent data and streamline data collection, storage, and processing, resulting in comprehensive and accurate datasets that support decision-making.
3. Intricate Regulatory Compliance
Manually tracking and updating compliance requirements is time-consuming and prone to errors, making it difficult for businesses to stay within regulatory boundaries.
Solution: Leveraging AI and ML solutions can ensure accuracy and timeliness in analysing compliance reporting and documentation, reducing the risk of non-compliance
4. Operational Inefficiency
With AI and ML, businesses witness an increase in operational costs and manual consumption of routine tasks in a larger amount of time, resulting in faster processes that reduce overall business productivity.
Solution: Integrating AI and ML into business operations can automate routine tasks, identify potential bottlenecks, streamline workflows, and save costs while improving performance and efficiency.
Did you know? The global machine learning market is forecast to reach $225.91 billion by 2030.
The statistical report shows that the adaptability of machine learning solutions will be essential for businesses to stay competitive and innovative.
Difference Between AI and Machine Learning
Integrating AI and ML is not just a requirement but a strategic move for businesses to stay competitive in the present and prepare for the future. Understanding their distinctions is crucial for effectively leveraging their unique capabilities.
FACT: In the world of technology, AI comprises machine learning, but not all machine learning qualifies as AI.
Let’s dive deep into AI and ML criteria that include:
Aspects | Artificial Intelligence | Machine Learning |
---|---|---|
Intended Goal | The main goal of AI is to perform tasks that understand and mimic human behaviour. It includes reasoning, problem-solving, and executing sophisticated tasks independently. | The major goal of ML is to ensure that machines can learn from data and make decisions and predictions without explicitly being programmed for each task. |
Methodological Approaches | AI implements many methods that are based on predefined rules to solve problems. This is created based on human behaviour, which is scalable and adaptable to different environments. | ML creates models and algorithms that can be fine-tuned from experience.
It primarily involves techniques like supervised, unsupervised, and reinforcement learning to make accurate prediction |
Conceptual Relationship | AI is a vast concept, and ML is just one of its subsets. It builds systems that can solve complex issues. | ML operates within the framework of AI, but its focus is narrower and depends on statistical models for specific tasks. |
Operational Workflow | AI functions as an adaptive model that enables natural language and utilises neural networks and statistics to make decisions and draw conclusions. | Machine learning functions by learning from data and training models to identify patterns. If the results are negative, the ML model is retrained. |
Development Process | Focuses on creating intelligent systems capable of autonomous decision-making. | Focuses primarily on algorithms that are trained and refined using large datasets. |
Key Applications | Example: AI is used in robotics, autonomous vehicles, virtual assistants (e.g., Siri, Alexa), smart home devices, and healthcare diagnostics. | Example: ML is used in banks to detect real-time fraudulent activities. It is also helpful in product recommendation and facial recognition. |
Implementation Needs | Implementing AI systems requires precise planning with high-quality data. The selected AI model should be able to automate repetitive learning and solve complex tasks. | Implementing ML models requires the right data capture, which needs to be prepared. Then, based on business requirements, the right model needs to be chosen and trained to make data-driven decisions. |
Resource Requirements | To implement and maintain, it required an effortless AI system, robust IT infrastructure, high computation power, large-scale data analysis, and well-trained AI experts. | An effective ML model requires fewer resources than AI. However, competent datasets are required to train and deploy the ML model. |
System Integration | Scalability, interoperability, and frequent updates are involved to ensure seamless integration with various technologies and systems | Real-time processing, datasets from diverse formats and APIs are required in deploying the ML models into the existing systems of a business. |
Scope of Adaptability | Various industries and domains have integrated AI systems that foster everything from simple automation to complex problem-solving. | Adaptable to environments where continuous learning and training from data are processed. |
Leverage custom dashboard & visualization solutions to transform data into actionable insights for streamlined operations and improved business decisions.
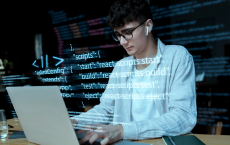
Industry Use Cases for AI and Machine Learning
Across industries, AI and ML have provided and continue to provide diverse support, changing how businesses operate and providing fresh perspectives. AI helps businesses automate tasks, analyse data, and improve decision-making. Machine learning provides cost savings, competitive advantages, and enhances customer experiences.
Let’s explore some of the use cases of AI and ML across industries
1. Banking Sector
AI solutions enhance the banking sector by offering real-time detection support by analysing transaction patterns through ML algorithms to improve security and prevent fraudulent activities.
Also, AI features are deployed in anti-money laundering software to detect suspicious transactions, identify patterns of illicit behaviour, and enhance regulatory compliance.
For example: Due to personal and security reasons, you want to deactivate the international usage of your credit card. But it’s late at night. Instead of waiting until the bank opens in the morning, you simply open your banking app and use the AI-powered chatbot.
The chatbot quickly understands your request, verifies your identity, and instantly deactivates your card’s international usage without waiting for business hours to finish the process.
2. Finance Services
AI and machine learning financial services are crucial, particularly in trading analytics, where large financial datasets, including market trends and trading volumes, are analysed to make informed investment decisions.
AI improves risk management by sending alerts for unusual financial activities, personalising banking services, and automating credit decisions and customer interactions. It transforms financial operations, increasing efficiency and forecasting trends.
For example: A hedge fund firm uses machine learning algorithms to forecast stock price movements by analysing historical trading data and economic indicators. The result? The firm makes smarter investments and drives higher returns by using data to guide its decisions.
3. Insurance
AI has transformed the insurance industry by analysing customer data or policyholders to personalise policies. Also, with the help of AI chatbot development, it offers 24/7 services for customers to solve queries regarding policies and claims. This reduces insurance companies’ operational costs and also fosters customer loyalty.
The manual data entry process can lead to many errors and create compliance issues. Automating the process with AI systems can mitigate these issues, reduce the risk of errors, and simplify the handling of complex tasks.
For example: You are applying for health insurance to cover your knee operation expenses. AI underwriting analyses your health-related documents to create personalised plans tailored to your needs. Whether you need preventive care, specialist consultations, or emergency coverage, AI ensures accurate risk assessment and benefits that suit you.
4. Telecommunication
The telecom industry is changing its landscape in new technological dimensions to improve customer service and experience. It used to focus only on mobile network services and telephones but now offers many products and services. Now, with a wide range of products and services, the telecom industry faces increased competition, requiring AI algorithms to stay ahead.
AI optimises telecom operations through ML and NLP, enhancing customer experiences and boosting revenue. Automated insights and proactive maintenance address big data management and network issue prediction. AI-driven solutions ensure efficient resource allocation and compliance, vital for telecom’s competitiveness and service excellence.
For example: AI can predict network issues before they happen, which improves network realibility, and use chatbots for instant customer support to foster customer loyalty.
5. Healthcare
Integrating AI into healthcare is not just an innovation but a transformation that optimises the patient experience and reshapes the medical landscape. Since everything is digital, AI systems analyse electronic health records to assess patient health and predict risks such as diabetes and heart attacks using machine learning models.
This approach produces faster and more accurate results, which facilitate proactive treatment plans and improve overall healthcare delivery.
For example: A wearable device monitors heart rate and alerts users to abnormal rhythms, potentially indicating health issues like atrial fibrillation (AFib). This early warning allows for timely medical intervention, reducing the risks associated with undiagnosed heart conditions
6. Manufacturing
Due to the complexity of production processes, extracting more data and making informed decisions is crucial in the manufacturing industry. It requires real-time insights to optimise efficiency and quality control. Major challenges occur in this industry due to process inefficiencies, errors, and high maintenance costs stemming from manual processes and delayed insights.
AI and ML algorithms are transformative choices for predicting equipment failures through sensor data analysis. This proactive approach optimises production efficiency by reducing costs and downtime through timely maintenance scheduling.
In automotive production units, ML algorithms analyse spare parts data to forecast demand, manage inventory efficiently, and cut costs. This will assist manufacturers in optimising supply chain operations and ensuring the timely availability of critical components.
For example: A leading retail store integrates artificial intelligence solutions to improve its supply chain operations. It also encompasses ML models to analyse sales data and predict customer demand, which are used for data-driven decision-making.
This initiative ensures retail store owners understand product availability, reduces stockouts, and optimises inventory costs, enhancing overall efficiency and customer satisfaction.
7. E-commerce & Retail
E-commerce is a prominent industry worldwide, and it faces new impacts daily due to technological transformations that open up new opportunities. AI and ML are at the forefront and have the potential to revolutionise the retail industry.
Reports indicate that 65% of consumers believe businesses that employ artificial intelligence. This means that ML and AI technology can improve customer perception and satisfaction.
Data mining allows for the collection of historical and current data to make effective predictions. Many enterprises gather data, but decision-making can be problematic, sometimes succeeding and other times failing. Machine learning solutions can help businesses leverage data to solve potential problems and make better decisions.
For example: When a formal dress is being searched for, an AI-driven visual search can be used to upload a picture of the desired dress. ML algorithms analyse the image and then provide details about similar products that are for sale on e-commerce sites, along with their prices. These innovations enhance pricing strategies and search capabilities.
8. Education
When it comes to education, AI and machine learning, including virtual and augmented reality, hold an immense position. These innovations help learners make the learning process more interesting and fun and contribute to modern education.
AI and ML technology upgrade the education industry by automating administrative tasks and the grading process, providing personalised learning experiences and data-driven insights.
For example: With the help of AI, content creation becomes easier, more advanced, and smarter for both teachers and students.
Teachers can create customised learning materials based on curriculum requirements to simplify and improve teaching effectiveness. They can also monitor their students’ performance using machine-learning capabilities and provide them with specialised learning materials based on that knowledge.
Students can access AI-powered learning apps and platforms for interactive learning experiences, multilingual learning support, and instant feedback to rectify errors.
9. Real Estate
AI and ML have transformed the real estate industry, modernising how properties are bought, sold, and invested in. AI solutions streamline the customer experience and property management, benefiting both businesses and consumers.
With the rise of brokers listing fraudulent property images to manipulate and cheat customers, AI and ML algorithms now detect such fraudulent activity, ensuring only valid property images are permitted for viewing by potential buyers, thereby increasing trust in the real estate industry.
For example: AI-powered real estate platforms offer many benefits, including a smooth process of buying, selling, and investing options, tailored property recommendations, automated property valuations, and an improved user experience.
10. Public Services
The usability of ML and artificial intelligence solutions has poised government operations by fostering public service activities such as document processing and solving customer queries more efficiently.
Inefficient processes like handling large amounts of data and manual paperwork create work delays. Rule-based AI-enabled software and related AI solutions can automate these manual and routine tasks. This automation could significantly improve government workflow and reduce backlog delays.
AI and machine learning algorithms help officials manage traffic flow, prevent accidents and congestion, and enhance road safety through data-driven analyses.
For example: AI-based language tools are leveraged to convert parliamentary information into digital formats. This includes making debates, questions, business agendas, and other content available in various languages, ensuring it’s accessible and understandable to everyone.
11. Legal Services
AI and ML technology offer fundamental benefits across industries, including automated routine tasks, boosted productivity, and lower operational costs. This approach is now being applied to legal services.
In legal processes, AI integration helps eliminate the challenges of managing and locating documents, reducing paperwork. Intelligent content management software, utilising AI and machine learning services, enables law firms to efficiently organise, collect, and access documents. This saves time, ensures compliance and protects the client’s sensitive information.
AI tools enhance legal services by assisting with e-discovery, legal research, document management, and due diligence, enabling lawyers to process large volumes of information quickly and accurately.
For example: AI-powered workflow tools help legal services progress with the required functional platform. They simplify contract drafting, improve legal research, and expedite document searches, improving productivity and fostering legal practices.
These tools provide strategic insights, help analyse briefs and litigation trends, and ultimately save time, enabling lawyers to focus on high-value tasks.
12. Tourism
AI has transformed many industries, including travel and tourism. It makes it easier for travellers to explore more places across the globe. AI also eliminates upgraded booking options, eliminating the need for travel agencies.
Key benefits of AI in the travel industry:
- Personalised travel options tailored to individual preferences.
- Advanced AI algorithms for more accurate travel recommendations.
- Increased comfort and convenience for travellers through AI-driven customer services.
For example: Trip advisory applications and websites use AI chatbots to effectively solve customer inquiries and needs. AI solutions allow travellers to book flights, make accommodations, and hire vehicles online through AI algorithms on social media platforms, providing an advanced and convenient booking experience.
Maximize customer engagement, streamline tasks, and provide flawless user experiences with innovative AI Chatbot Development Solutions for business success.
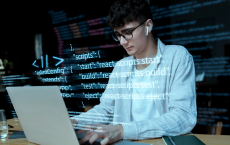
Steps to Implement AI and ML Solutions
Successfully implementing AI and ML solutions in enterprises may seem challenging, but with careful planning and execution, it proves to be highly effective.
The benefits of machine learning and artificial intelligence across industries include predictive analytics, personalisation, and an enhanced customer experience. Their transformative potential for the business landscape has driven innovation and efficiency.
1. Define the Goals & objectives
The first step before implementing AI and ML solutions is to start with “WH” questions to develop a clear strategy to understand your business goals.
- What specific problems or opportunities does your business have that AI and ML can address?
- Why are AI and ML implementation important for our business strategy?
- Which business goals would you like to achieve (e.g., customer experience, prediction of market trends, business operations) with AI and ML solutions?
- Where should AI and ML solutions be implemented within our organisation?
- How will you measure business success in AI and ML initiatives?
Answering these questions sets a clear path for your business to set effective goals and objectives.
2. Ensure Organisational Capability
Once the organisation’s goals are identified, the next step is to understand the infrastructure and tools that will support AI and ML initiatives. Leveraging high-performing servers and cloud-based resources to handle large datasets.
Data is the key to success for the effectiveness of AI and ML in an enterprise. Ensure that the data inputted into AI and ML models is accurate, relevant, and secure, which is vital for producing reliable and insightful results.
Consider using platforms such as TensorFlow, PyTorch, Scikit-Learn, and Spark MLlib to create and implement machine learning models. This would facilitate organisations’ full use of data lakehouse power.
3. Reach Out for Expert Assistance
The third and most important step is to choose AI and machine learning experts who are capable of implementing these twin technologies.
- Analyse whether they have essential knowledge of AI and ML, including processes of deep learning and natural language processing.
- Explore the expert’s portfolio of work on AI and ML projects and case studies to proficient and track record in delivering successful implementations.
- Request proposals to review their detailed plans, strategies, and pricing for project implementation to determine whether they are reasonable and meet your organisation’s goals.
- Arrange a meeting and communicate with AI and ML professionals to grasp their approach to the project and their method for providing updates.
- Ensure AI experts prioritise fairness, data privacy, transparency, and ethical policies in their development practices.
Looking for professional AI and ML solution implementation? SquareOne Technologies is one of the most reputable digital transformation companies offering expert ML and AI consulting services in the Middle East for successful implementation and ethical development practices.
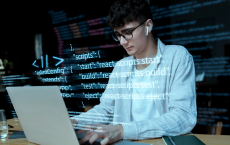
4. Understand the Business Use Case
The question that directly aligns with the step of analysing and understanding the business use case for AI and ML integration is
“What problems or opportunities does your business have that AI and ML can address?”
The challenges include improving business operations using AI to manage resources, make decisions faster, and automate repetitive tasks. AI and ML can also predict future issues, making operations more flexible and fostering organisational innovation
5. Recommend the Right Solution for Business
Analyse the best available AI and ML tools and pick the right solution. Consider choosing AI—and ML-enabled software and solutions that can be customised and prepacked.
It includes enhancing document management, streamlining workflow automation, effectively managing big data, and more customisations aligned with business needs to achieve rapid wins and maximise ROI.
The primary goal is to choose tools that align closely with your unique business goals. They must also meet your specific requirements and address challenges effectively.
6. Deploy the AI and ML solutions
After addressing all your goals, objectives, and challenges and finding the right AI and ML-based solution provider, it’s time to integrate these solutions into existing systems or deploy new AI-powered platforms within your organisation.
7. Run, Test and Monitor
After implementing artificial intelligence and machine learning solutions, start using them gradually in a phased manner.
It includes
- First, the solution can be tested with a small pilot project or each department.
- Second, monitor and gather feedback, fix potential issues, make improvements and continue the flow with the remaining departments.
- Continuously monitor the performance of the AI and ML solutions and maintain them regularly.
- This phased approach helps keep the process smoother and more effective, ensuring successful implementation and continuous improvement in business operations.
8. Post Implementation Support
- Facilitates continuous tracking of deployed AI and ML models’ performance to ensure they deliver the desired outcomes.
- Provide ongoing training and support for end-users to maximise their ability to utilise AI and ML tools effectively.
- If the AI and ML solution encounters a challenge or issue, immediate assistance will be provided, ensuring smooth operation and minimal disruption to business processes.
- Conducts regular surveys to gather user feedback and insights and facilitates continuous improvement to avoid queries or problems and maximise the benefit of machine learning and AI for future business operations.
SquareOne's Strategic Implementation of AI and ML Solutions
SquareOne, a prominent digital transformation company, is recognised as a reliable provider of AI and machine learning solutions in the Middle East. Our team of experts harnesses the power of data to deliver innovation and foster exceptional business results for our clients. We understand the core capabilities of AI and machine learning, including data analysis, NLP, predictive modelling, adaptive algorithms, and automation.
At SquareOne, our professionals leverage AI and ML solutions to address possible business requirements and potential challenges. These solutions efficiently handle large data volumes, provide future insights, and optimise business processes, leading to significant cost savings and possible outcomes.
Final Note
Embracing artificial intelligence and machine learning empowers businesses to innovate and thrive in a data-driven world. These technologies are one-stop solutions equipped with continuous upgrades for businesses to stay ahead of the curve.
Are you still struggling to navigate the intricacies of AI and ML? Let SquareOne be your trusted partner in providing digital transformation consulting in the Middle East. We ensure the seamless integration of AI and ML-enabled software solutions into your business, guaranteeing the achievement of goals through
- Advanced Deep Learning Techniques
- Cost-effective Acceleration of Efficiency
- Reliable Automated Operation Processes
- Supervised Algorithm Assurance