Data Enrichment 101
A Step-by-Step Guide to Enriching Your Data
KEY TAKEAWAYS
- Reliable data sources serve as the foundation for enriched data that ensures accuracy.
- Data enrichment involves cleaning and validating existing data to guarantee its validity and completeness.
- B2B Enterprises gain an advantage in the market by empowering them to make better marketing choices with b2b data enrichment application and techniques
Introduction
In today’s business landscape, data is as solid as rock to gather insights for making strategic choices and increasing customer experiences. However, the challenge arises when large volumes of collected data lack quality and functionality.
To truly benefit from data, it needs to be cleaned up and organized. Without a refined data, even the most advanced analytics tools won’t deliver the expected results.This is where data enrichment becomes crucial, unlocking the full potential of data by adding quality and additional insights.
Data enrichment is a process that helps businesses enhance their current data by adding relevant information, thereby improving accuracy and reliability. This process aids in making better decisions and strengthening relationships with customers.
Explore the essential chapters of this blog, covering the understanding, techniques, innovations, benefits, and challenges of data enrichment to upgrade customer insights and drive business growth.
Understanding Data Enrichment
1. Purpose of Data enrichment
Adding value to the current datasets is the main purpose of data enrichment, which helps organizations achieve their business data enrichment goals.
Here is an in-depth understanding of the purpose of data enrichment:
- Advancing Customer InsightsWithout knowing who your customers are, it is challenging to make tailored marketing plans and strategies and deliver personalized experiences. Data enrichment bridges this gap, by offering meaningful insights that add value to the current customer data. This data includes the customer’s preferences and purchase history to create a detailed view of customers.
For Example : In a retail store, an indepth knowledge of customers can be better achieved by creating a customer profile that includes a list of frequent shoppers, regular purchase histories of products (likes and dislikes). With this enriched data, businesses can make superior decisions, increase awareness about products that customers prefer, and improve customer engagement.
Ultimately, this leads to increased customer satisfaction, loyalty, and retention, driving long-term business success with tailored strategies.
- Increasing Data AccuracyEnhancing data correctness is paramount for companies and is achievable through the integration of algorithmic matching with third-party systems. This approach validates and updates existing data comprehensively, rectifying errors, filling in missing details, and eliminating duplicate records.
With these measures, businesses can ensure data integrity, optimize operations, and make informed choice-making.
- Improving Data Relevance Neglecting to leverage relevant data can negatively impact business efficiency. Data enrichment plays a critical role in strengthening the relevance of data by amplifying its quality, depth, and usability through the integration of additional information from various sources (geographic information, industry-related topics).
To guarantee that data remains relevant, focus on clear goals before collecting, gather selectively, and use real-time methods.
For Instance : Financial institutions collect data pertaining to geopolitical events, industry trends, and real-time financial metrics, augmenting their current datasets with additional insights. This enables them to better comprehend market dynamics, make accurate predictions, identify extensive opportunities, and mitigate potential risks.
Through the enrichment of the data, financial institutions enhance their decision-making processes, ultimately optimizing investment strategies and delivering value to their clients.
- Enabling Long-Term Growth Having enriched data can help organizations find possible opportunities for growth and innovation. Moreover, companies not only stay ahead of the curve and understand market trends but also create the framework for long-term expansion and competitiveness in the dynamic business environment.This method is useful for B2B businesses since it allows them to explore their knowledge of potential emerging markets and develop innovative products and services.
- Adapting to Evolving Market Dynamics
Staying informed about industry-related shifts and developments is essential for unlocking your business’s growth potential. Data enrichment facilitates this process by enabling businesses to monitor market dynamics and adapt to prevailing trends effectively.
2. Types of Data Enrichment
Data enrichment acts as a transformative tool, converting your existing data into comprehensive profiles about your customers. It amplifies the value and usability of data by refining and enhancing its quality.
Today, organizations utilize various methods of data enrichment to enhance their datasets, aiming to refine and improve raw data.
Let’s delve into the types of data enrichment:
- Demographic EnrichmentAdding information regarding the personal details of the customer includes age, gender, and occupation, helps companies personalize targeted marketing campaigns and product/service offerings that drive higher engagement rates and conversions.
For Example : An online ecommerce store could analyze age-related data to tailor marketing campaigns and product recommendations, optimizing for the most active age groups to boost sales and engagement.
- Geographic Enrichment
Adding information related to geographic data, including postal codes, country and city, to the current data sets enhances the data value. Geographic data helps to target the maximum number of audiences from a specific geographic area, plan location-based marketing campaigns, or determine the best locations to open new stores, ensuring optimal reach and engagement.
For Instance : A restaurant chain uses geographic enrichment to analyze customer addresses and identify clusters of loyal customers in certain areas. By doing so, they can target these regions with special promotions and decide where to open new branches. This strategy helps the restaurant chain optimize its marketing efforts and expand efficiently into high-potential locations.
- Behavioral Enrichment
Behavioral enrichment, also known as purchase intent enrichment, involves analyzing a customer’s purchase data. This enrichment provides brands with valuable insights into both past and present buying behaviors, enabling them to make informed decisions and tailor their marketing strategies effectively.Example: purchase history, engagement patterns and website interaction.
- Psychographic Enrichment
Psychographic enrichment involves capturing the behavioral patterns, personal interests of customers, and personalities that include lifestyle attributes.Example: Values, hobbies, personal opinions
- Technographic Enrichment
Technographic data provides insights into the technological tools and platforms utilized by your potential clients. This includes understanding their use of hardware and software applications and their emerging automation strategies. Companies may improve their marketing and sales efforts by using this data to better understand their current technological platforms and forecast what their customers will want in the future when it comes to purchasing technology.
For Instance : Technographic data reveals the tech tools a company employs, from software like CRM and project management systems to hardware preferences and industry-specific tools. This intelligence guides businesses in customizing solutions and outreach to match their prospects’ tech landscape and preferences.
3. Role of Data Sources for Enriching Data
The success of obtaining enriched data relies on sourcing information from credible data sources. Then, what exactly is a data source?
The data source serves as the point of origin for the data that businesses use. Initially, data was physically derived, but with technological advancements, data sources have transitioned to digital platforms, facilitating the acquisition of refined and digitized data.
Data sources are essential for enriching data, as they
- Supply pertinent and high-quality data to augment the usefulness, precision, and depth of raw data.
- Allow for data integration and validation, ensuring uniformity and clarity.
- Adhere to applicable laws and regulations, upholding data privacy and governance standards.
Two types of data sources are
- Internal Data Sources
Information collected from and utilized within the company’s domain is known as internal sources. Internal data assists organizations by offering insights regarding operations, analyzing performance and driving business decisions.This type of data is mainly extracted from internal departments, which include marketing, sales, customer support, finance and HR, and streamlines analysis to identify areas for improvement and take the required action to foster performance. - External Data Sources
Information collected from third parties, known as external sources, provides additional insights for the benefit of company success. Industry trends, competitor achievement, and new opportunity identification are just a few examples of how external sources enrich businesses’ perspectives.
Importance of Data Enrichment
Augmented data gives you deeper insights into your company’s operations and customer base, making it easier to increase your brand’s visibility and impact. That’s why data enrichment is essential, as it provides the additional insights necessary for a deeper understanding of your targeted users in your business.
In this process, data extraction serves as the initial step, retrieving raw information from diverse sources. Then, this raw data is scrubbed and improved through validating, normalizing, and appending extra characteristics. Data enrichment boosts the original dataset, enhancing its depth, precision, and usability.
With an enriched dataset sourced from multiple channels, organizations can make more informed decisions, foster customer experiences and facilitate growth through actionable insights.
Note: “The more enriched data you have, the more strategic decisions can be taken swiftly.”
Let’s breakdown the importance of data enrichment
Improving Data Quality
Accuracy and reliability are the cornerstones of data enrichment, which improves the quality of the data, whereas data quality observability plays a pivotal role in an organization’s operations. It involves the process of monitoring data integrity to certify trustworthiness for deeper analysis and crucial decision-making processes.
Inaccurate data can result in inefficient allocation of advertising funds, diminished customer satisfaction, and flawed analytical outcomes, posing significant costs for companies. Many B2B businesses keep unnecessary data because they don’t know it’s there or aren’t sure what to eradicate.
Note: B2B data enrichment ensures the quality of extracted data is errorless and trustworthy through transcribing, completing, and refining.
Market Expansion Opportunities
Businesses grow and expand to grab new market opportunities through data enrichment, which requires a holistic approach with the necessary data. These data were extracted from a wide array of data sources offering demographics, consumer behaviors, and prevailing trends within the target market.
Businesses utilize sophisticated data extraction tools to gather information and employ data enrichment techniques that enhance the quality and depth of the data, offering valuable insights. This active process contributes to ongoing success in exploring new market opportunities.
According to the Data Integrity Trends and Insights Report:
- Only 46% of executives trust the data they use for decisions.
- Over 40% of respondents struggle to address data standardization and verification.
Data enrichment processes address these challenges by enhancing data precision through robust enrichment processes. It can enhance the quality of data by standardizing and verifying it against reliable sources.
Improved Decision Making
Data enrichment plays an important role by customizing information to align with the unique requirements of a business. Effectively understanding and managing data is essential for thoughtful decision-making.
By incorporating additional context, the data becomes more precise and complete. This enriched data forms a robust basis for insightful analysis, leading to more effective and successful outcomes. Improved data quality and relevance enable businesses to make strategic choices that foster growth and maintain a competitive edge.
Competitive Advantage
Businesses gain a competitive edge in today’s market landscape with the help of data enrichment. Data enrichment aids industries in mitigating risks by providing a complete picture of consumer behavior and purchase history, driving better risk management.
In post enrichment, businesses leverage data to easily conduct competitive studies, measuring feasibility, which helps them find new opportunities and remain feasible.
Moreover, to increase engagement and conversions, data enrichment allows businesses to effectively gain prospective customers and divide lead enrichment according to their level of interest in the brand.
Note: “Data enrichment boosts competitiveness through personalized marketing and tailored products that help businesses better meet customer needs.”
Dos and Don’ts of Data Enrichment
The process of data enrichment is to optimize the quality of datasets with additional information from the various channels, which aims to make them more accurate and reliable.
For business owners, data analysts, marketing, sales, and finance professionals, it’s crucial to grasp what practices to embrace and what to avoid when it comes to data enrichment before implementation.
Here are the actions and pitfalls to steer clear of:
Dos
-> Understand your Objective Clearly
Establish precise goals before initiating data enrichment to certify alignment with business objectives and desired outcomes.
-> Prioritize Data Quality Over Quantity
Focus on obtaining data that maintains relevancy and adds value to the enrichment process rather than gaining a larger volume of data with insufficiency and missed information.
-> Verify the Credibility
Validate the authenticity of data sources to maintain the integrity of enriched data.
-> Ensure Legal Compliance
Adhere to data protection laws ( GDPR and CCPA) and regulations to safeguard the privacy rights of individuals or organizations during the enrichment process.
-> Conduct Pilot Tests Before Integration
Before full integration, conduct trial runs or pilot tests to assess the effectiveness, identify potential issues and refine the enrichment process.
Don’ts
-> Avoid Complicating Datasets
Simplify datasets by excluding unnecessary variables or data points that do not contribute any valid points to the enrichment objectives, which could complicate the enrichment process.
-> Never Ignore Data Privacy Regulations
Mandate collecting information with consent by maintaining compliance with data privacy regulations to uphold the rights and privacy of individuals, which drives trust and credibility.
-> Don’t Overlook the Data Documentation Process
Records of the data enrichment process verify transparency, accountability, and replicability, facilitating troubleshooting and future improvements. So avoid neglecting the process of data documentation.
-> Avoid Over-Reliance on Automation Tools
While automation tools can streamline the enrichment process, human oversight is essential to validate results, identify errors, and certify quality control.
Learn more about advanced Enterprise Content Management solutions that efficiently manage cloud content and processes, governance to boost productivity, minimize errors, and meet regulatory requirements
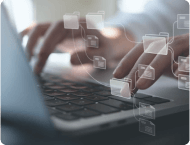
Data Cleansing
What is Data Cleansing
Data cleansing, also called data scrubbing or cleaning, is the process of identifying and fixing errors, inconsistencies, and quality issues in data to make it more accurate and reliable.
Data cleansing improves data quality by removing duplicate data, correcting errors, and standardizing data to ensure it is credible and easier to understand. This results in informed business decisions and successful outcomes.
Differences Between Data Cleansing and Data Enrichment
Data enrichment and data cleansing serve two different purposes. Enrichment focuses on adding additional information from various sources to the current datasets to add value, whereas cleansing focuses on improving the data by removing duplicates and correcting errors.
Both data cleansing and enrichment helps businesses increase the significance and accuracy of data through
- Enabling Better Insights and Decisions
- Improving Operational Efficiency
- Establishing a Reliable data source
Reminder: “The common aim of data enrichment and cleansing is to provide trustworthy data that can help companies make better decisions and run more efficient operations.”
Let’s break down the differences between data cleansing and data enrichment:
Aspect | Data Enrichment | Data Cleansing |
---|---|---|
Purpose | Adding additional information from external sources that is relevant to the current datasets. | Removing excess, inaccurate, or irrelevant information to maintain factualness. |
Goal | Enhances the data’s effectiveness and depth by incorporating valuable insights, improving its overall usefulness and solidity. | Ensures the given data is spot-on, reliable , and complete. |
Business Impact | Empowers businesses to gain deeper insights, personalize marketing efforts, and make informed decisions. | Improves operational efficiency, and decision-making accuracy by providing clean and authentic data. |
Examples | Adding location and job title information to an existing email list. | Deleting fake email addresses and duplicate contacts from a marketing database. |
Note: “Data cleansing refines data by correcting errors, while enrichment enhances its data quality observability by adding supplementary information.”
Techniques of Data Enrichment
A data enrichment technique refers to the specialized methods and processes employed to enhance and improve the quality, value, and usefulness of existing data. This involves augmenting the primary data set with additional information from external or internal sources, providing complete comprehension, and facilitating more effective choices.
Accurate decision-making and strategic planning rely on high-quality data, which helps mitigate risks like inaccuracies, data silos, and compliance issues. Data enrichment plays a significant role in maximizing the value of data assets by enhancing their quality and utility.
Let’s break down the techniques of data enrichment:
- Data Segmentation
Data segmentation is the process of dividing data into distinct categories according to predetermined criteria to facilitate better analysis and to take informed actions. To understand customers better, data segmentation is pivotal for businesses to optimize customer data and analytics for fostering customer loyalty. Businesses can tailor these segment criteria based on their goals and requirements.
For Instance : A bank segments its customers based on their income level, credit score and spending details; it offers personalized financial services and loyalty programs that include credit cards with high interest rates and higher cashback rates during specific purchase activities.
Examples of data segmentation includes
- Demographic: Adds personal details to customer profiles.
Example: age, gender, and occupation. - Geographic: Includes the data of where customers live.Example: country, city, or zip code.
- Firmographic: Adds information about customers’ companies.Example: Industry sector or company size
- Behavioral: Shows how customers behave and interact.Example: purchase history, engagement patterns and website interaction
- Technographic: Reveals the technology that customers use. Example: device usage, software preferences, and digital adoption trends.
- Psychographic: Provides insights into customers’ interests and personalities that include lifestyle attributes.
Example: interests, values, hobbies, personality, opinions
To put it simply: “Data segmentation involves dividing large datasets into smaller, well-defined groups, allowing for targeted analysis.”
- Data Imputation Data imputation is the process of adding a different value to substitute a missing value to retain the majority of the information in the dataset. This technique guarantees that analyses remain precise and reliable despite gaps in the data. Regularly removing data from a dataset is unfeasible, so the imputation technique is integrated to maintain the dataset’s completeness and reliability.
Data imputation is crucial for several key reasons.
- Imputation maintains dataset completeness, essential for valid statistical analysis, particularly in smaller datasets.
- It also enables the use of machine learning models that require complete data to function correctly.
- Moreover, imputation helps meet research and industry standards for acceptable levels of missing data, making datasets more robust and comparable.
- Additionally, it reduces the need for additional data collection, saving time and resources.
Techniques of data imputation
- Mean Data imputation utilizes the mean, a simple and effective technique that replaces missing values with the average value from the entire dataset.
- Mode Mode is employed to replace frequent missing values (numerical and categorical data) in the dataset, helping to maintain the most common attribute within the dataset
- Median Median is particularly useful for numerical data and is employed to replace missing data with a median value in the dataset, as the median is less affected by extreme values compared to the mean.
- Regression Regression predicts missing values using a regression model trained on the available data to predict the missing values based on other related features. This method leverages relationships between variables to provide a more accurate imputation.
- K- Nearest Neighbor K- Nearest Neighbor (KNN) method is a machine learning algorithm used to solve both classification and regression problems. Data imputation includes this technique to find missing values that have similar instances in the dataset.
- Data Apending Data appending is the practice of filling in missing information within a given database. It implies enriching existing datasets by incorporating additional data from external sources. This process enhances the depth and context of the dataset, contributing to a more comprehensive understanding of the information at hand. Moreover, costs associated with data are minimized, which simplifies operations while generating insightful data that fuels business development. Data appending is essential for businesses as it certifies the correctness and completeness of their databases. It enhances the consistency of business data by filling in the missing information, facilitates better customer interactions, tailors their products and services, and improves overall operational efficiency.
- Data Categorization Data categorization demands classifying data into different categories or classes based on its sensitivity, relevance, and intended audience. The primary objective is to guarantee that data is handled, stored, and shared in a manner that aligns with its importance and confidentiality level. Data categorization is a fundamental aspect of modern data management practices, and its significance lies in its ability to verify data security, streamline operations, and facilitate regulatory compliance.
Benefits of Data Enrichment
Data enrichment is not a one time investment; it is a continuous process that helps business grow in the long run. Businesses can get more out of their data by enriching it, which helps them understand things better, drive better choices, and make changes that improve their operations and the customer experience.
Here are some of the key benefits of data enrichment:
Improved Data Quality
To maintain solidity and trustworthiness, incorporating a data enrichment tool effectively analyzes information and eliminates any misleading, biased, and inaccurate data, ensuring reliability of data insights. Data enrichment is a technique that can improve the quality of your data by adding new information to your existing datasets.
Companies might face a lot of challenges, including ineffective advertising, choices of action, and customer dissatisfaction due to data quality issues that are flawed or deceived. Data enrichment applications and their tools can mitigate or eradicate these challenges effectively, thereby accelerating business expansion and elevating customer expectations.
Informed Decision-Making
A well informed decision in an organization can be taken with the help of qualitative datasets. It means that the company can analyze the given data and expand the scope of integration with additional external data sources.
Data enrichment facilitates businesses to uncover more additional insights and connections, which helps to take more strategic decision-making that match organizational goals. Businesses can utilize enriched data to discover fresh patterns, insights, helping your organization stay current, relevant, and competitive.
Streamlined Risk Management
Companies get a detailed picture of ongoing operations, customer preferences and market conditions with the help of data enrichment. It enables companies to understand and find potential issues and wearly, so they can take proactive steps to manage and mitigate risks effectively.
Cost-Effectiveness
Choosing quality over quantity when it comes to data can result in reducing the unknown costs spent on data storage and the high volume of datasets. Businesses spend money on larger datasets without understanding the value of the data.
However, with data enrichment, businesses can enhance data accuracy and current data sets and cut costs by retaining only the most pertinent and impactful data essential for the business.
Applications of Data Enrichment for Enterprises
After reading the meaning, importance, and benefits of data enrichment, you might get an understanding of what data enrichment is. Simply put, data enrichment involves refining and enhancing existing datasets with additional data to make them more valuable.
Now let’s comprehend the applications of data enrichment and how enterprises leverage data enrichment to optimize their operations and services. It includes different techniques and processes that are used to improve the quality, depth, and usability of governing data within organizations.
Exploring the applications of data enrichment, which include:
1. Market Intelligence for Strategic Expansion
With the help of data enrichment, businesses have the ability to assess the behavior of their competitors, determine their target markets, and capitalize on chances for expansion with market intelligence. This advantage gives businesses the ability to investigate high-potential areas of growth with the help of various instruments.
Strategic expansion is nothing but setting up a vision with a preplanned roadmap and long term goals for getting into new market areas. Data enrichment helps enterprises gain a clear understanding of the target market, which includes interests, preferences, and behaviors, as well as assessing market dynamics and competition.
2. Optimizing Sales and Marketing Efforts
Data enrichment plays a pivotal role in optimizing sales and marketing endeavors. By equipping teams with essential information like company size, industry, and job titles, it facilitates precise customer segmentation. This segmentation enables personalized content delivery, enhancing engagement and satisfaction levels among leads and customers.
Data enrichment streamlines lead qualification processes by filtering out irrelevant data, thus improving the efficiency and effectiveness of sales efforts.
On the marketing and sales front, data enrichment can boost lead generation and assist in improving conversion rates. With enhanced sales data, marketing and sales teams can optimize their strategies to yield better results.
3. Enhancing Customer Experience
Consumer data enrichment serves as a handy tool for enterprises to enhance the customer experience, which helps in getting deeper insights into their preferences, behaviors, and needs to attain targeted customer groups.
84% of service leaders prioritized customer data and analytics for organizational goals.
This underscores the significance of data enrichment in shaping customer experiences, allowing businesses to tailor services and interactions based on consumer data enrichment.
With an enriched customer profile (demographic information, purchase intentions, interests, and brand preferences), businesses can offer personalized strategies, offerings and interactions to meet individual customer expectations.
This tailored approach fosters stronger connections with customers, leading to increased satisfaction, loyalty, and advocacy.
4. Personalization
Enriched customer data drives fundamental personalization in creating memorable customer experiences. By leveraging data enrichment, enterprises can streamline their communication across multiple channels (emails, social media, and websites) by tailoring personalized messages and addressing large customer pain points.
Personalization strategies involves
- Maintaining regular engagement with customers,
- Addressing their pain points precisely
- Offering solutions that cater to their specific needs.
Data Enrichment for Various Industries
Data enrichment is a powerful tool used across different industries to change how businesses use data. It helps them come up with new ideas, enhance customer experiences, and gain competitive advantages. From finance to retail to beyond, data enrichment applications and their techniques empower companies to unlock valuable insights, optimize operations, and stay ahead in an ever-evolving marketplace.
Let’s explore how data enrichment is reshaping industries worldwide:
- 1. Retail and Ecommerce
By 2024,retail e-commerce sales are expected to surpass 6.3 trillion U.S. dollars globally, signifying the indispensable role of e-commerce in the retail sector. This means having authentic customer data enables businesses to implement strategic marketing approaches and deliver efficient services tailored to customer needs.
Effective data management is crucial for success in e-commerce. Integrating product information management (PIM) with generative AI offers a smart product data enrichment solution. This combination streamlines processes, automates content creation, and enhances personalized customer experiences.Generative AI helps automate tasks (data analysis, tagging multimedia content, predictive maintenance) and businesses can improve data efficiency and maintain consistency across channels. As technology evolves, embracing this integration will keep e-commerce driving sales and staying ahead of the curve.
- 2. Financial InstitutionsAn advancement in data enrichment involves creating solutions that can effectively mitigate risks and detect fraud promptly.
When it comes to financial institutions, data enrichment is indispensable in upgrading financial products and services through open banking data to create more comprehensive customer profiles.
In digital onboarding and KYC, data enrichment verifies customer identities through external sources like government databases, reducing fraud and improving communication precision.
In payment processing, data enrichment validates payment details and assesses the risks associated with different payment types. Enriched data uses history and geolocation data to monitor real-time transactions and detect unusual patterns and possible fraud. - 3. Banking SectorData enrichment in banking fosters an advanced mode called payment enrichment data that heightens fraud detection by adding required insights to payment transactions. It allows banks to assess risks, analyze customer behavior, and identify variations effectively.Improved detection safeguards customer accounts, protects the bank’s financial interests and integrates the role of enriched data in modern banking security.
For Instance : With enriched data in payment services, the banking sector can strengthen fraud detection through the blending of data like geolocation and transaction activities. This helps banks pinpoint the risks better and secure bank accounts, which maintain reliability and trust among stakeholders.
- 4. Government Sectors
Data enrichment helps the government by collecting and organizing lots of different information from many places in the country. This helps the government understand things better, like how to give better services, be clear and fair, and react better to emergencies.
For Example : Data enrichment can assist the government in analyzing confidential matters regarding the country and tracking information about those sensitive issues to find solutions and make clear decisions.
Learn more about our unified and Intelligent content service platform for seamless data capture, automated workflows, and accurate document management, optimizing businesses, operations
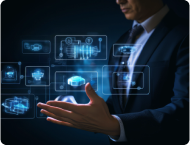
Challenges of Data Enrichment
Extracting raw data from external sources, refining it and adding it to existing datasets to increase its current value is the goal of data enrichment. It offers massive advantages, including greater segmentation and audience targeting. However, without facing any challenges, it is impossible to fully utilize the power of data enrichment solutions.
Explore the challenges of enriching data for businesses:
- Data Quality and AccuracyThe more qualitative information you have on your customers, the better you can understand them and make their interactions easier. This approach fosters the customer relationship and results in more sales.Incorrect, outdated, or incomplete data can result in poor decisions and strategies in the implementation of tailored solutions for the target audience.
It is vital to ensure the trust and credibility of both existing and new data. This involves regular checks and validation to uphold the data quality strategy and prevent errors.
For Example : A sales team uses enriched customer data to tailor their sales pitches. If the data contains outdated phone numbers, their outreach efforts will be wasted, resulting in missed opportunities and reduced customer conversion.
- Scalability and PerformanceQualified and enriched data empowers businesses to enhance their performance. When the volume of data increases, the storage of data becomes a major issue. Many businesses collect information from various channels but struggle to maintain a well-equipped database to store such information.
This happens due to budget constraints and outdated systems. As data continues to expand, choosing the right data enrichment solutions becomes crucial to seamlessly scaling with evolving data needs and ensuring reliability. - Handling Large Volumes of DataLarge datasets include diverse data types, formats, and structures, and maintaining data quality can be quite challenging, which leads to data errors. It requires a calculative system with computing performance, storage space, memory, and processing speed to process and enrich data effectively.
- Data Consistency and Standardization
Standardization involves converting data into a uniform format because a large volume of data is collected in various formats. So storing that data can be a complex process. Lack of consistency in data sources causes errors and inaccuracies in the augmented dataset.
Also, it’s crucial to regularly check and fix data quality issues to avoid problems with consistency. By doing this, enterprises can prevent issues with standardization and ensure that your data stays accurate and reliable.
For Example : A company’s dataset contains inconsistencies in the format of country names, with some entries recorded as “Saudi Arabia” and others as “KSA,” which results in inconsistency and requires maintaining uniformity and enrichment in the data. This dataset requires standardization and is needed to enable more effective analysis for various business purposes.
- Data Privacy and Security
Adhering to privacy regulations such as GDPR and CCPA is essential in data enrichment, which involves the process of collecting and storing large amounts of sensitive data. Failure to fully encrypt or protect this data can result in data breaches and legal consequences for enterprises.
Steps to Implement Data Enrichment for Your Organization
Implementing data enrichment in an organization enhances data truthfulness and quality by incorporating relevant information. This process helps organizations stay competitive, maximize the value of their datasets, and build greater trust with their customers.
Three main reasons to implement data enrichment includes
- Strengthen the quality and value of the data by adding additional information and filling the missing gaps.
- Data enrichment boosts lead qualification and routing by pinpointing and prioritizing high-value leads, reducing the need for manual research and data entry.
- Insights offered into the ideal customer profile facilitate targeted marketing, personalized outreach, and optimized sales approaches.
Enriched data can significantly boost ROI by improving customer outreach and increasing conversion rates in marketing campaigns. While data enrichment might seem challenging initially, it is a straightforward and highly effective process.
Effective implementation ensures data is reliable and actionable, improving consumer experiences and business objectives.
Steps to implement data enrichment are:
- Establish Clear Data GoalsUnderstand and analyze your business requirements. Define specific objectives for data enrichment, such as improving customer profile authenticity and increasing marketing strategies with measurable and achievable targets.
- Assess Data Quality Evaluate the completeness, quality, and loyalty of your existing data to identify any gaps or inconsistencies before starting the enrichment process. Build and test the data quality and observability to analyze, cleanse, and enrich the data.
- Leverage Enrichment toolsInvest in a powerful data enrichment tool to streamline the collection, organization, cleansing, formatting, and enrichment of data to enhance efficiency and completeness. These tools automate repetitive tasks, allowing your team to focus on more strategic initiatives and ensuring that your data is reliable and consistently up-to-date.
- Segment your audienceTarget and restrict data sets based on specific audience segments. Guarantee that the enrichment activity is tailored to the unique needs and preferences of each group, which improves relevancy
- Prioritize Data PrivacyEnsure that your data enrichment processes comply with legal standards and prioritize user privacy to maintain trust and compliance with data protection laws.
- Keep Data UpdatedContinuous updating and enrichment of your data is essential. Since data enrichment is a continuous process, it’s critical to keep the data upgraded and validated in order to guarantee its accuracy and applicability over time.
Note: To keep ahead of data deterioration, make regular schedules for data cleanup and invest in automated enrichment solutions to remain spot-on and up-to-date.
Future Trends of Data Enrichment
The future of data enrichment promises to revolutionize how businesses extract insights, prevent fraud, and optimize operations in an era defined by unprecedented data volumes and technological advancements
Artificial Intelligence and Machine Learning
Data enrichment with artificial intelligence (AI) and machine learning (ML) facilitates advanced analysis and predictive insights, which qualify swift processing of data, reduce time and cost, and validate the identification of intricate patterns and trends in real time.
For example, a fashion retailer can leverage AI and ML to automatically generate tailored outfit suggestions and personalized style advice for customers based on their previous purchases and browsing habits, thereby enhancing customer engagement and driving sales.
Blockchain for Data Verification
Accuracy and reliability are the major components of data enrichment when blockchain technology fuses in; it provides a decentralized approach to data validation and enhances the value and trustworthiness of data. Blockchain ensures data integrity and transparency, particularly in industries where data security is paramount, such as finance and healthcare.
IoT Integration
As the Internet of Things (IoT) continues to expand, data enrichment will play a vital role in extracting valuable insights from the vast amount of data generated by connected devices. By enriching IoT data with additional contextual information, businesses can optimize operations, improve supply chain logistics, and enhance predictive maintenance efforts.
For example, wearable devices in healthcare, such as smartwatches and Fitbits, gather data on users’ physical activity, heart rate, and sleep patterns. By combining this data with external information like medical history and environmental factors (e.g., air quality and pollen levels), it allows tailored health recommendations, early detection of potential health issues, and overall improved patient outcomes.
Marketing and Sales Optimization
Businesses can leverage data enrichment to gain deeper insights into their customers, enabling personalized marketing campaigns, targeted advertising, and improved customer segmentation to boost engagement and conversion rates.
Through the use of data enrichment, you can add crucial information about your leads, resulting in a considerably more accurate score for each lead and driving conversions. Businesses can effectively identify and convert these leads by obtaining additional details, such as company email addresses, job titles, and locations.
Enrichment with Privacy as Priority
Data privacy is known to be an essential concern that will continue to escalate in the long run. Enrichment services are emphasizing methods that comply with privacy regulations and reduce data quality issues, gaining prominence for their security.
Techniques such as differential privacy and federated learning are being explored to ensure data privacy while still enabling valuable insights from enriched data.
Final Note
Data enrichment improves data quality strategy by integrating external information or enhancing existing datasets, leading to more robust and valuable insights. It adds quality and depth to the data, helping businesses adapt to evolving customer and industry trends.
Neglecting the continuous enrichment process means industries are missing out on opportunities to deliver relevant offers and experiences to their customers, and personalization.
With the right enrichment solutions, techniques, and strategies, organizations can foster innovation, competitiveness, and success. Data enrichment integrates advanced technological solutions, including AI, ML, and blockchain, to enhance data efficiency, solidity and integrate real-time monitoring capacities.
Explore more at the forefront of data enrichment innovations and fortify your organization with enriched and secure datasets for future business endeavors.
Dive into our blogs to learn more about data enrichment: